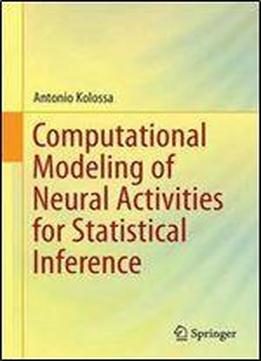
Computational Modeling Of Neural Activities For Statistical Inference
by Antonio Kolossa /
2013 / English / PDF
13.3 MB Download
Authors: Kolossa, Antonio
Provides empirical evidence for the Bayesian brain hypothesis
Presents observer models which are useful to compute probability distributions over observable events and hidden states
Helps the reader to better understand the neural coding by means of Bayesian rules
This authored monograph supplies empirical evidence for the Bayesian brain hypothesis by modeling event-related potentials (ERP) of the human electroencephalogram (EEG) during successive trials in cognitive tasks. The employed observer models are useful to compute probability distributions over observable events and hidden states, depending on which are present in the respective tasks. Bayesian model selection is then used to choose the model which best explains the ERP amplitude fluctuations. Thus, this book constitutes a decisive step towards a better understanding of the neural coding and computing of probabilities following Bayesian rules. The target audience primarily comprises research experts in the field of computational neurosciences, but the book may also be beneficial for graduate students who want to specialize in this field.
Number of Illustrations and Tables
22 b/w illustrations, 20 illustrations in colour
Topics
Mathematical Models of Cognitive Processes and Neural Networks
Biomedical Engineering
Neurosciences
Physiological, Cellular and Medical Topics
Simulation and Modeling