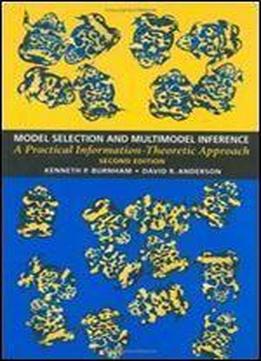
Model Selection And Multi-model Inference
by Kenneth P. Burnham /
2002 / English / PDF
4.5 MB Download
The second edition of this book is unique in that it focuses on methods for making formal statistical inference from all the models in an a priori set (Multi-Model Inference). A philosophy is presented for model-based data analysis and a general strategy outlined for the analysis of empirical data. The book invites increased attention on a priori science hypotheses and modeling. Kullback-Leibler Information represents a fundamental quantity in science and is Hirotugu Akaike's basis for model selection. The maximized log-likelihood function can be bias-corrected as an estimator of expected, relative Kullback-Leibler information. This leads to Akaike's Information Criterion (AIC) and various extensions. These methods are relatively simple and easy to use in practice, but based on deep statistical theory. The information theoretic approaches provide a unified and rigorous theory, an extension of likelihood theory, an important application of information theory, and are objective and practical to employ across a very wide class of empirical problems. The book presents several new ways to incorporate model selection uncertainty into parameter estimates and estimates of precision. An array of challenging examples is given to illustrate various technical issues. This is an applied book written primarily for biologists and statisticians wanting to make inferences from multiple models and is suitable as a graduate text or as a reference for professional analysts.